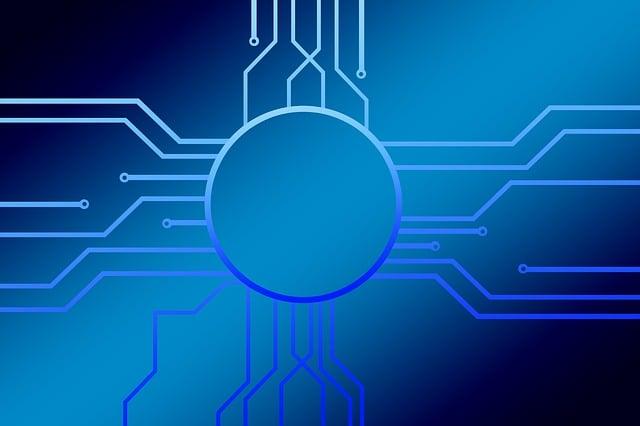
High product quality is the lifeline of any manufacturing company and this aspect will make or break the brand. Yes, quality matters, and only by paying keen attention to it can organizations achieve a good reputation with customers coming back for more. Issues related to quality such as errors due to manual inspection, use of outdated technology, poor maintenance of equipment, and worn-out calibration tools will lead to quality defects.
It is always safe to invest in inspection rather than facing the huge costs of possible defects or breakdowns. Yes, the implementation of a robust quality inspection strategy is essential to earn customers’ trust, and business continuity and gain a competitive edge in the marketplace.
How is it done today? Challenges with conventional machine vision
Conventional vision systems operate via step-by-step filtering and rule-based algorithms. The rules have to be logically sequenced and programmed on a case-to-case basis, where each rule will have multiple configurable parameters. These systems excel at the quantitative measurement of a structured scene because of their speed and accuracy. However, this machine vision will find it rather difficult to comprehend and function in complex cases. The systems struggle to handle variability and deviation between very visually similar parts where algorithms become unwieldy as exceptions and defect libraries grow leading to performance issues.
How can this be re-imagined? AI-based visual inspection overcomes the challenges of conventional systems
Deep learning which is a subset of Artificial Intelligence (AI) teaches machines to learn by example. AI fits in multi-layered deep neural networks that mimic neuron networks in the human brain where the machine vision systems learn from them and enhance their inspection competencies. They now can recognize images, distinguish trends and make intelligent predictions and decisions. They continuously refine performance as they are presented with new images and can distinguish unacceptable defects while tolerating natural variations in complex patterns.
The key advantage of this method is the end-to-end learning process. Furthermore, there is no need of defining the features and perform feature engineering as the neural network has the capability of doing that by itself. This approach is called data-driven modeling, where a neural network is taught to identify a feature or a defect, and this can be achieved by showing images of that specific feature.
A real-world example - Quality Control aspects across a typical Auto supply chain
To get a better picture of Quality Control in a supply chain, let us take the example of the Auto manufacturing sector. The raw material stage to the component manufacturing is done across 10 steps. The next assembly stage has 500 components and there is a 15% loss between these two stages. From the Assembly to OEM Stage which consists of 40 assemblies that manufacture 30,000 components, there is a 1-2 % loss.
In each step of the supply chain, there are three stages for quality inspection that include incoming, in-process, and final. At each stage, two aspects of Quality Control (QC) are addressed which include, measurable defects, where the quality regarding size and number can be assessed objectively, and cognitive defects that revolve around visual, audio, and identification. In the latter, due to the enormous variety, there is no objective way to assess the total quality of 100%.
Across the supply chain, there is a need for 100% real-time assurance in cognitive QC and not just sampling and it is important to make traditional machine vision work to leverage investments made. Insights driven balance between economic and quality levers is required to make this economically viable. Priority should also be placed on scaling silo-ed SME knowledge to reduce employee fatigue.
Opportunity to re-imagine Quality Control
AI-enabled QC solutions are today extensively leveraged by manufacturers and assemblers, OEMs, Warehouses, and the logistics industry. Surface and Audio defect detections, and their presence or absence are done at the component manufacturers and assemblers. OEMs perform inspections for components received from their suppliers. Additionally, the package and pallet inspection and traceability aspects are much needed for warehouses and the logistics sector.
With the AI-enabled visual inspection, these industries benefit significantly with 98% accuracy in defect detection, a 30-40% reduction in false rejections, a 15% reduction in PPM (parts per million), and a 20% increase in speed of inspection. The return on investment in this modern AI-enabled visual inspection solution is around 12 to 18 months.
The many factors to achieve success in re-imagining QC are well-defined defects, understanding and designing hardware and automation, choosing the right approach and data to train models, using data to continuously improve model accuracies, and more importantly having patience as it is an iterative process.
Other aspects to be taken into consideration for the transformation include building in-process and end-of-line inspection automation, using analytics from defect detection to improve processes, and integrating product traceability, adding to Industry 4.0 ecosystem.
Edge AI and Next-gen for Quality Control are gaining traction
The combination of Edge Computing and AI, which is Edge AI addresses the latency issue of data being sent to solutions in the cloud and data centers. Power consumption is less with no issues in runtime. The algorithms are processed on the machines at the assembly lines or a server that is nearby. Decisions are faster without the data traveling to and from the cloud or the internet. Deployment of Edge AI-enabled visual inspection will be high across assembly lines for the many benefits it delivers. Data is maintained within the organization and does not leave the company’s network.
For products with high variety and frequent design changes, instant learning which is a next-gen technology will be in demand. This next-gen AI Tech will deliver anomaly detection for unknown and unseen defects. Here AI “understands” what normal looks like and “imagines” it where the input image is “healed” to represent the OK component.
Automotive, food and pharma, general manufacturing, warehousing and logistics, printing, and electronics are some of the industry verticals that have successfully adopted AI and Edge technologies for their quality inspection processes.
Add new comment